Martin Rabe, Stefan Milz, Patrick Mäder
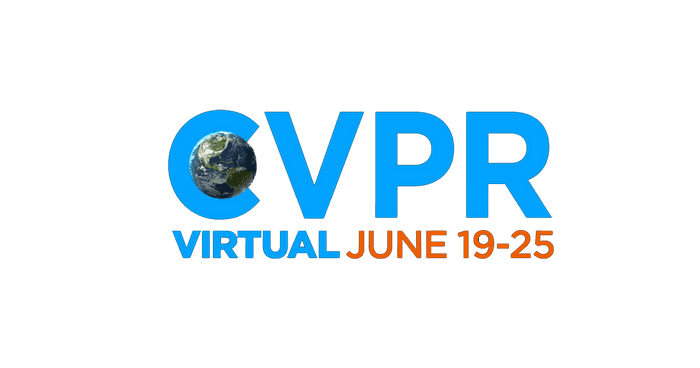
Abstract: Enabled by recent advances in the field of machine learning, the automotive industry pushes towards automated driving. The development of traditional safety-critical automotive software is subject to rigorous processes, ensuring its dependability while decreasing the probability of failures. However, the development and training of machine learning applications substantially differs from traditional software development. The processes and methodologies traditionally prescribed are unfit to account for specifics like, e.g., the importance of datasets for a development. We perform a systematic mapping study surveying methodologies proposed for the development of machine learning applications in the automotive domain. We map the identified primary publications to a general machine learning-based development process and preliminary assess their maturity. The reviews’s goal is providing a holistic view of current and previous research contributing to ML-aware development processes and identifying challenges that need more attention. Additionally, we list methods, network architectures, and datasets used within these publications. Our meta-study identifies that model training and model V&V received by far the most research attention accompanied by the most mature evaluations. The remaining development phases, concerning domain specification, data management, and model integration, appear underrepresented and in need of more thorough research. Additionally, we identify and aggregate typically methods applied when developing automated driving applications like models, datasets and simulators showing the state of practice in this field.
